Note
Go to the end to download the full example code.
Sample slices from volumes¶
In this example, volumes are padded, scaled, rotated and sometimes flipped. Then, 2D slices are extracted.
import matplotlib.pyplot as plt
import torch
import torchio as tio
torch.manual_seed(0)
max_queue_length = 16
patches_per_volume = 2
subject = tio.datasets.Colin27()
subject.remove_image('head')
subjects = 50 * [subject]
max_side = max(subject.shape)
transform = tio.Compose(
(
tio.CropOrPad(max_side),
tio.RandomFlip(),
tio.RandomAffine(degrees=360),
)
)
dataset = tio.SubjectsDataset(subjects, transform=transform)
patch_size = (max_side, max_side, 1) # 2D slices
def plot_batch(sampler):
queue = tio.Queue(dataset, max_queue_length, patches_per_volume, sampler)
loader = torch.utils.data.DataLoader(queue, batch_size=16)
batch = tio.utils.get_first_item(loader)
fig, axes = plt.subplots(4, 4, figsize=(12, 10))
for ax, im in zip(axes.flatten(), batch['t1']['data']):
ax.imshow(im.squeeze(), cmap='gray')
plt.suptitle(sampler.__class__.__name__)
plt.tight_layout()
Uniform sampler¶
When a torchio.UniformSampler
is used,
some of the patches don’t contain much useful information:
sampler = tio.UniformSampler(patch_size)
plot_batch(sampler)
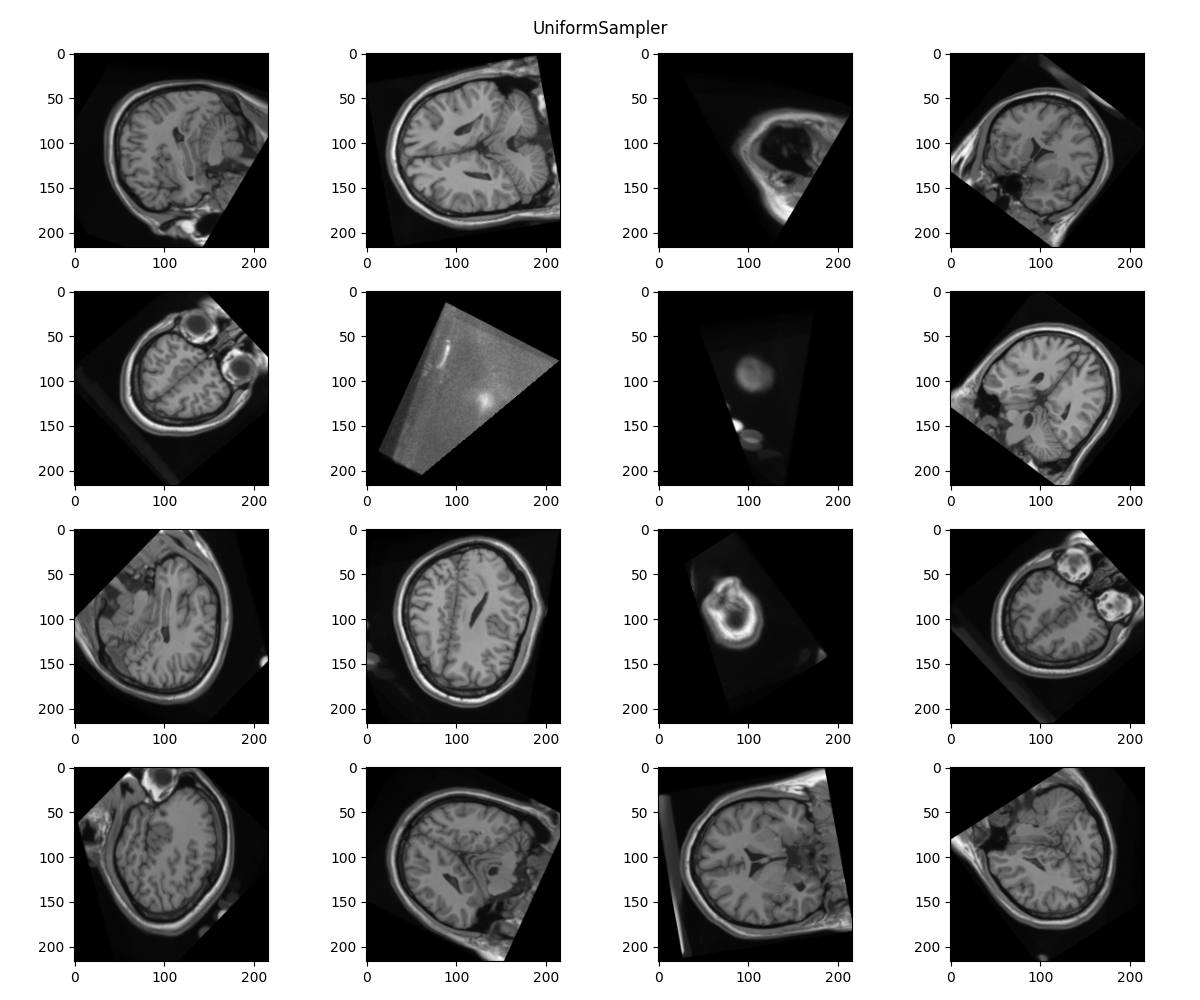
Weighted sampler¶
We can use the brain
image contained in the subject as a probability map
for a torchio.WeightedSampler
. That way, we ensure that the center
of all patches correspond to brain tissue.
sampler = tio.WeightedSampler(patch_size, probability_map='brain')
plot_batch(sampler)
plt.show()
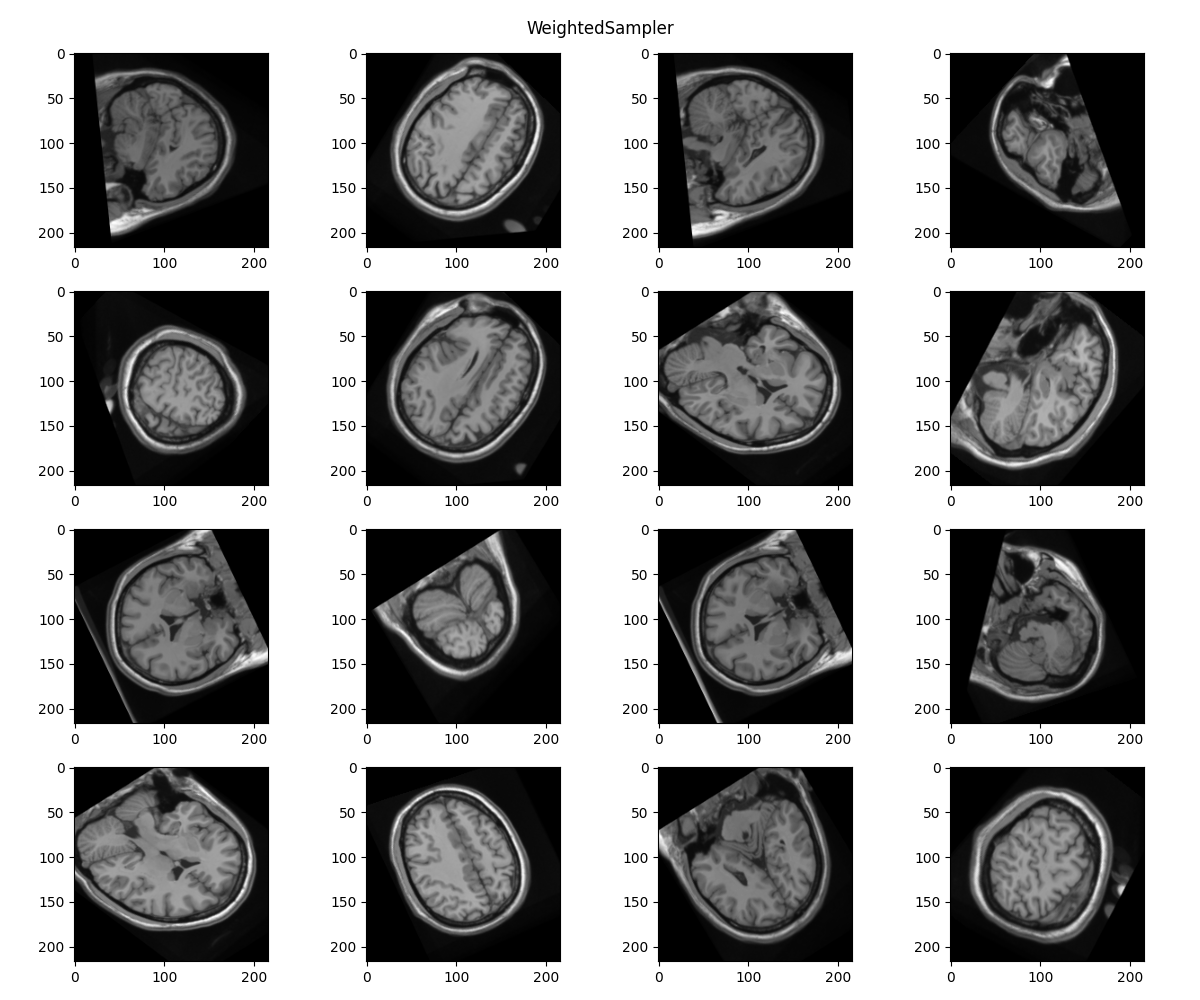
Total running time of the script: (0 minutes 22.680 seconds)